News and Updates
In reverse chronological order:
-
Feb. 2025: I received the ATK-Nick G. Vlahakis Graduate Fellowship!
-
Feb. 2025: SPARK paper submitted to RSS!
-
Jan. 2025: Safe control for quadrupeds paper accepted to ICRA, see you in Atlanta!
-
Dec. 2024: Our new safe humanoid toolbox, SPARK, is out!
-
Nov. 2024: Demo'ed F1Tenth vehicles at Safety21 University Transportation Center!
-
Oct. 2024: Started working on humanoids!
-
Sep. 2024: Safe navigation for package-carrying quadruped paper submitted to ICRA!
-
Jul. 2024: See you at ACC in Toronto!
-
Jun. 2024: Presented one paper at ECC in Stockholm!
-
Feb. 2024: Robust-adaptive controller paper accepted to ECC, see you in Sweden!
-
Jan. 2024: ModelVerification.jl paper submitted to CAV!
-
Oct. 2023: Robust-adaptive controller paper submitted to ECC!
-
Aug. 2023: I started my master's at CMU Mechanical Engineering!
-
Jul. 2023: I joined the Intelligent Control Lab @ CMU RI!
-
May. 2023: Graduated from UC Berkeley! See you in Pittsburgh!
-
May. 2023: Finished the EKF project for the Indy Autonomous Challenge!
-
May. 2022: I am joining Tesla as a Vehicle Dynamics / Software Engineering Intern!
-
Jul. 2021: I finished my 10-month internship as an RL Engineer at NeuroCore.ai!
-
Jan. 2021: Back to Berkeley after my military service in the Korean Army!
|
|
SPARK: A Modular Benchmark for Humanoid Robot Safety
Yifan Sun,
Rui Chen,
Kai S. Yun,
Yikuan Fang,
Sebin Jung,
Feihan Li,
Bowei Li,
Weiye Zhao,
Changliu Liu
Submitted to Robotics: Science and Systems (RSS), 2025.
arXiv | Website | Video
To alleviate the challenge of robust safety measures in general humanoid operation, we introduce the Safe Protective and Assistive Robot Kit (SPARK), a modular toolbox that integrates state-of-the-art safe control algorithms into a generic humanoid control framework.
SPARK enables users to configure safety behaviors across multiple dimensions, such as defining safety criteria and sensitivity levels, allowing for optimized trade-offs between safety and performance.
|
|
Safe Control of Quadruped in Varying Dynamics via Safety Index Adaptation
Kai S. Yun,
Rui Chen,
Chase Dunaway,
John M. Dolan,
Changliu Liu
Accepted to International Conference on Robotics and Automation (ICRA), 2025.
arXiv | Website | Video
We deploy Safety Index Adaptation (SIA) for a quadruped robot to safely navigate in varying dynamics. SIA enables real-time adaptation of safety indices to ensure provable safety.
With SIA, the quadruped carries packages of varying weights and sizes while navigating through obstacles without failure. Moreover, we introduce a novel linear model for varying quadruped dynamics and a method to identify the changing dynamics.
|
|
Synthesis and Verification of Robust-Adaptive Safe Controllers
Simin Liu*,
Kai S. Yun*,
John M. Dolan,
Changliu Liu
Published in European Control Conference (ECC), 2024.
IEEE | arXiv
We investigate controller synthesis for dynamical systems with uncertain parameters. We designed an optimization algorithm for generating robust-adaptive safe controllers
that can guarantee safety in the presence of uncertainties, without being overly conservative. Our controller performs 55% better compared to popular robust controllers.
|
|
ModelVerification.jl: a Comprehensive Toolbox for Formally Verifying Deep Neural Networks
Tianhao Wei,
Luca Marzari*,
Kai S. Yun*,
Hanjiang Hu*,
Peizhi Niu*,
Xusheng Luo,
Changliu Liu
Under review at International Conference on Computer Aided Verification (CAV), 2024.
arXiv | GitHub
We introduce a new comprehensive toolbox for formally verifying deep neural networks. ModelVerification.jl is a Julia package (with Python interface) that provides a wide range of
state-of-the-art verification algorithms for various deep neural networks. This toolbox is designed to be user-friendly and efficient, and it is open-source.
|
|
Mass-independent Dunham Analysis of the [7.7] Y2 Σ+ – X2 Πi and [16.3] A2 Σ− – X2 Πi Transitions of Copper Monoxide, CuO
Jack C. Harms,
Ethan M. Grames,
SirkHoo Yun,
Bushra Ahmed,
Leah C. O'Brien,
James J. O'Brien
Journal of Molecular Spectroscopy, 2019.
Journal of Molecular Spectroscopy
The previous literature on the electronic spectrum of Copper-63 Oxide is extended to Copper-65 Oxide. We combine the analysis of A-X and Y-X electronic
systems of Copper-65 Oxide, using the mass-independent Dunham fit with PGOPHER software to obtain molecular constants. Moreover, Copper-isotope field-shift is corrected to
the electronic exictation energy required in the fit of Y-X system.
|
|
Humanoid Teleoperation
Demo video
In this project, we developed a teleoperation system for Unitree G1 humanoid robot with Apple Vision Pro (AVP).
Using the AVP, the system tracks the human operator's hands and translates the end-effector poses into the humanoid's upper-body movements.
|
|
Balancing an inverted pendulum on quadrotor with LQR
Demo video
For this project, we implemented a Linear Quadratic Regulator (LQR) controller to balance an inverted pendulum on a quadrotor.
I built the quadrotor, named "Danaus-12", with PX4-Autopilot firmware. A carbon fiber tube is used as the pendulum and it is not mechanically
attached to the quadrotor. Instead, it simply rests on the quadrotor and the LQR controller is used to balance the pendulum.
The quadrotor is controlled with an offboard computer and I used a Vicon motion capture system to track the positions of the quadrotor and pendulum.
This work is based on "A flying inverted pendulum", by Markus Hehn and Raffaello D'Andrea from ETH.
|
Tesla, Inc., Vehicle Dynamics Team
Vehicle Dynamics / Software Engineering Intern • May 2022 to August 2022
|
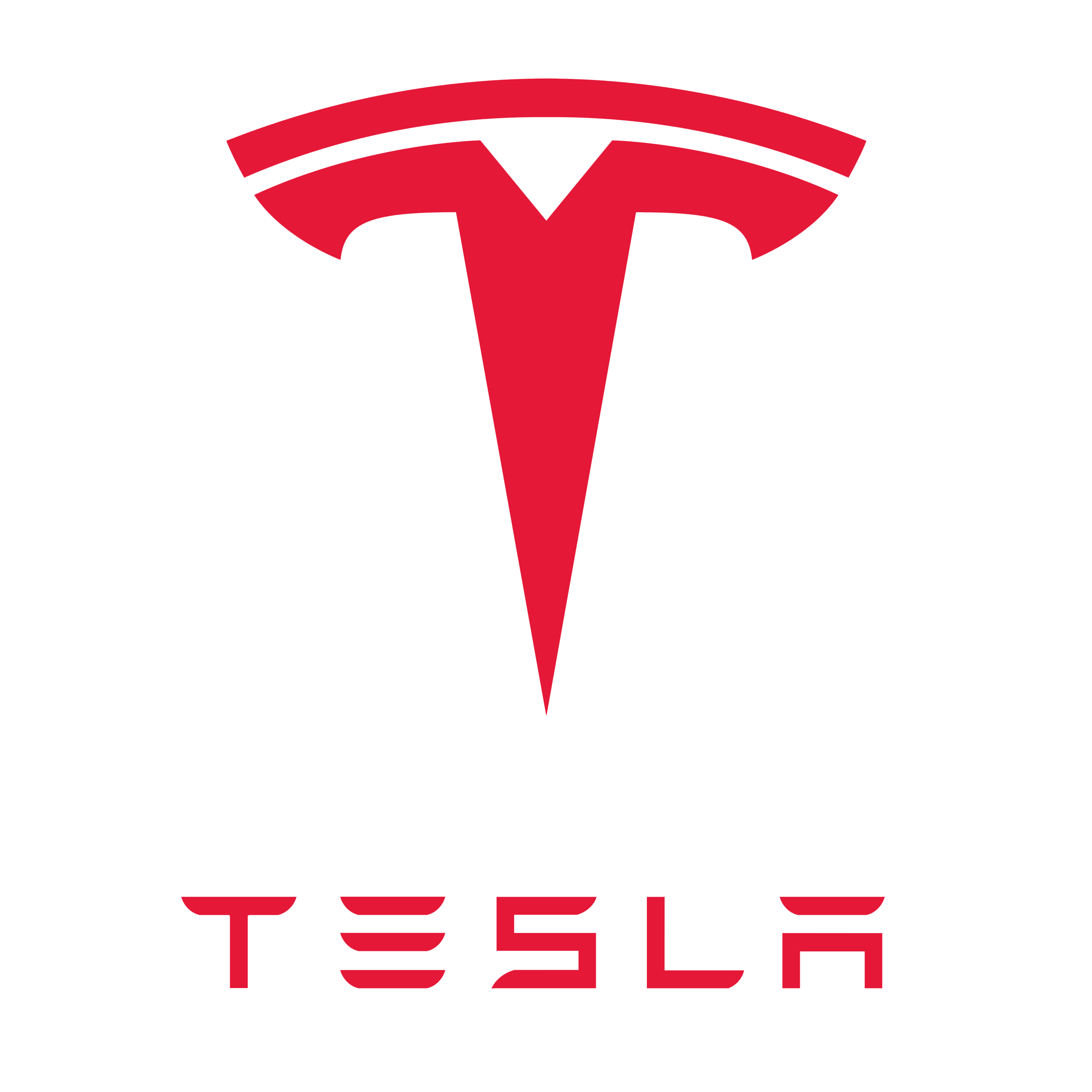 |
NeuroCore.ai., Reinforcement Learning Team
Reinforcement Learning Research Intern • October 2020 to July 2021
|
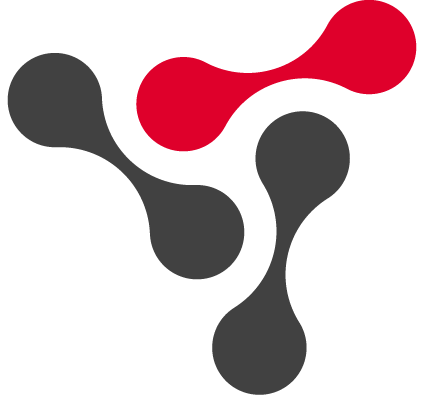 |
|